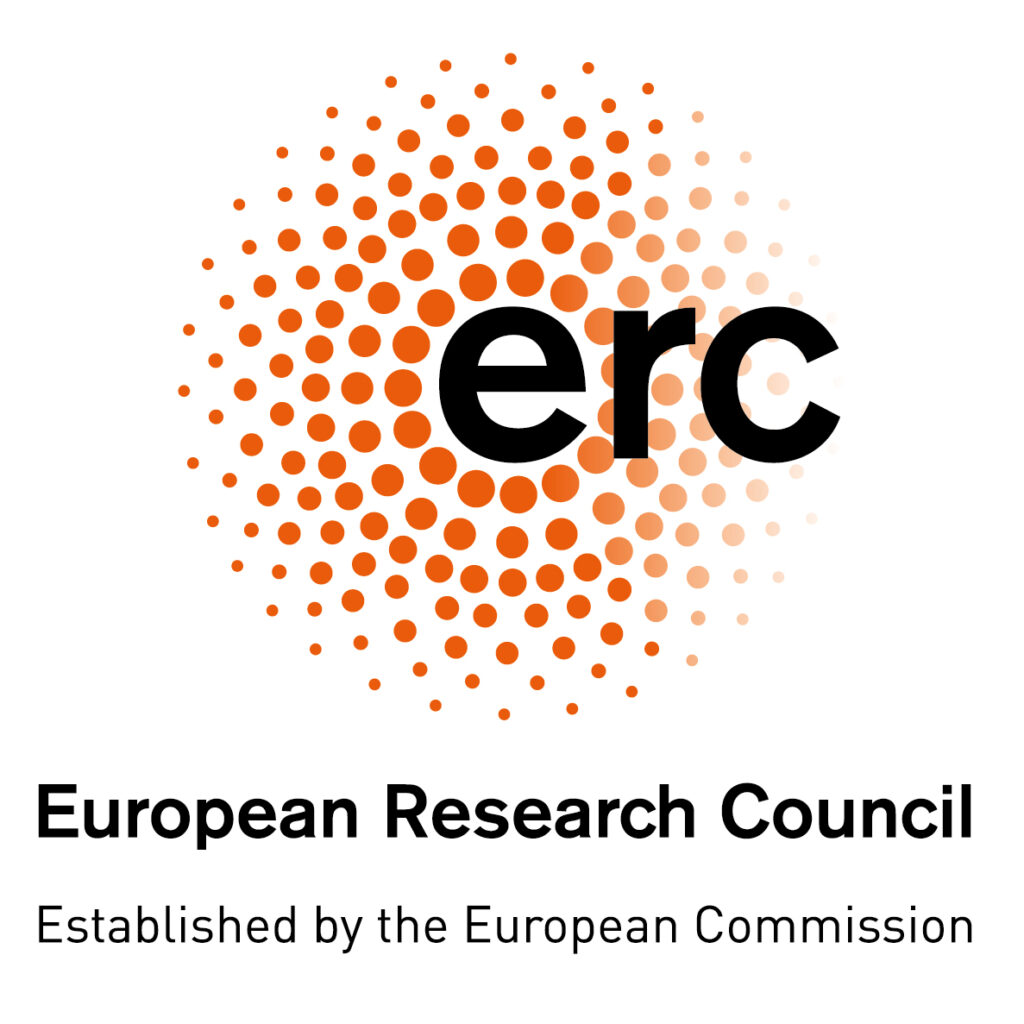
Classical neural networks, originally inspired by the neural structure of the brain, have become a powerful and ubiquitous information processing paradigm in our every day’s life. Neural-network-based algorithms and software are used with impressive success for tasks as diverse as image and speech recognition, machine learning, the analysis of ‘big data’ and ‘deep learning’. Driven by the hope of combining properties such as massive parallel information processing in neural networks with advantages like the computational speedup promised by quantum computers, there have been efforts in several directions to develop quantum neural networks.
The overarching goal of this theoretical research program, which started in October 2019, is to establish and explore a conceptual framework for quantum neural networks, based on concepts from open many-body quantum systems, and to identify quantum optical physical building blocks for the practical implementation of such a quantum processor paradigm. Thereby, the project aims at laying the foundation for quantum neuromorphic engineering of quantum neural hardware in state-of-the-art and newly emerging experimental systems, including trapped ions, Rydberg atoms and hybrid platforms.
In recent works [1,2] we proposed a new framework to understand how quantum effects may impact on the dynamics of neural networks. In particular, we developed open-system quantum generalisations of the celebrated Hopfield and Potts-Hopfield neural networks, simple toy models of associative memory, which allowed us to treat thermal and quantum coherent effects on the same footing.
[1] Open quantum generalisation of Hopfield neural networks, P. Rotondo, M. Marcuzzi, J. P. Garrahan, I. Lesanovsky, and M. Müller, Journal of Physics A: Mathematical and Theoretical 51, 115301 (2018).
[2] Phase diagram of quantum generalized Potts-Hopfield neural networks, E. Fiorelli, I. Lesanovsky, and M. Müller, arXiv: 2109.10140 (2021).
This research is supported by ERC Starting Grant QNets.